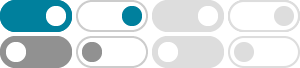
PMNN: Physical model-driven neural network for solving time …
2023年12月1日 · In this paper, an innovative Physical Model-driven Neural Network (PMNN) method is proposed to solve time-fractional differential equations. It establishes a temporal iteration scheme based on physical model-driven neural networks which effectively combines deep neural networks (DNNs) with interpolation approximation of fractional derivatives.
PMNN:Physical Model-driven Neural Network for solving time …
2023年10月7日 · In this paper, an innovative Physical Model-driven Neural Network (PMNN) method is proposed to solve time-fractional differential equations. It establishes a temporal iteration scheme based on physical model-driven neural networks which effectively combines deep neural networks (DNNs) with interpolation approximation of fractional derivatives.
In this paper, an innovative Physical Model-driven Neural Network (PMNN) method is proposed to solve time-fractional differential equations. It establishes a temporal iteration scheme based on physical model-driven neural networks which effectively combines deep neural networks (DNNs) with interpolation approximation of fractional derivatives ...
PMNN: Physical model-driven neural network for solving time …
2023In this paper, an innovative Physical Model-driven Neural Network (PMNN) method is proposed to solve time-fractional differential equations. It establishes a temporal iteration scheme based on physical model-driven neural networks which effectively combines deep neural networks (DNNs) with interpolation approximation of fractional derivatives.
PMNN:物理模型驱动的神经网络,用于求解时间分数阶微分方 …
本文提出了一种创新的物理模型驱动神经网络(PMNN)方法来求解时间分数阶微分方程。 它建立了基于物理模型驱动的神经网络的时间迭代方案,有效地将深度神经网络(DNN)与分数阶导数插值近似相结合。 具体来说,一旦分数阶微分算子被离散化,DNN 就被用作将插值近似技术与微分方程集成的桥梁。 在此集成的基础上,我们构建了基于神经的迭代方案。 随后,通过训练 DNN 来学习这种时间迭代方案,可以获得微分方程的近似解。 所提出的方法旨在尽可能保留方程中 …
GitHub - SummerLoveRain/PMNN_IPMNN
In this article, we propose two kinds of neural networks inspired by power method and inverse power method to solve linear eigenvalue problems. These neural networks share similar ideas with traditional methods, in which the differential operator is realized by automatic differentiation.
Papers with Code - PMNN:Physical Model-driven Neural Network …
2023年10月7日 · In this paper, an innovative Physical Model-driven Neural Network (PMNN) method is proposed to solve time-fractional differential equations. It establishes a temporal iteration scheme based on physical model-driven neural networks which effectively combines deep neural networks (DNNs) with interpolation approximation of fractional derivatives.
[PDF] PMNN: Physical Model-driven Neural Network for solving …
2023年10月7日 · In this paper, an innovative Physical Model-driven Neural Network (PMNN) method is proposed to solve time-fractional differential equations. It establishes a temporal iteration scheme based on physical model-driven neural networks which effectively combines deep neural networks (DNNs) with interpolation approximation of fractional derivatives.
[2310.04788] PMNN: Physical Model-driven Neural Network for …
In this paper, an innovative Physical Model-driven Neural Network (PMNN) method is proposed to solve time‑fractional differential equations. It establishes a temporal iteration scheme based on physical model-driven neural networks which effectively combines deep neural networks (DNNs) with interpolation approximation of fractional derivatives.
PMNN: Physical model-driven neural network for solving time …
2023年12月1日 · In this paper, an innovative Physical Model-driven Neural Network (PMNN) method is proposed to solve time-fractional differential equations. It establishes a temporal iteration scheme based on physical model-driven neural networks which effectively combines deep neural networks (DNNs) with interpolation approximation of fractional derivatives.