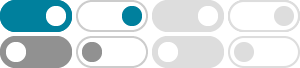
Title: Mini-AOD: A New Analysis Data Format for CMS - arXiv.org
2017年2月15日 · The CMS experiment has developed a new analysis object format ("Mini-AOD") targeting approximately 10% of the size of the Run 1 AOD format. The motivation for the Mini …
[1702.04685] Mini-AOD: A New Analysis Data Format for CMS
The CMS experiment has developed a new analysis object format (”Mini-AOD”) targeting approximately 10% of the size of the Run 1 AOD format. The motivation for the Mini-AOD …
699元确实香 华米Amazfit GTS 2 mini评测:经典方屏全能小清新
在Amazfit GTS 2 mini智能手表的身上,我们还体验到了全面的运动健康功能,除了传统的心率、血氧、睡眠监测外,还有华米特色“PAI健康评估系统”,为职场用户提供了全面直观的健康监 …
Mini-AOD: A New Analysis Data Format for CMS - IOPscience
2015年12月1日 · The CMS experiment has developed a new analysis object format ("Mini-AOD") targeting approximately 10% of the size of the Run 1 AOD format. The motivation for the Mini …
For these reasons, a new compressed data format called Mini-AOD has been developed. Mini-AOD is 10% of the size of AOD, and it replaces the multiple intermediate datasets used in Run 1.
Packed PF Candidates in Mini-AOD For all packed candidates some basic info is saved: ━ PDG ID, 4-vector, charge, impact parameters ━ Lossy compression applied on variables, with …
Mini-AOD: A New Analysis Data Format for CMS - ResearchGate
2015年12月23日 · In this contribution we discuss the critical components of the Mini-AOD format, our experience with its deployment and the planned physics analysis flow for Run 2 based on …
Mini-AOD: A New Analysis Data Format for CMS - CERN …
The CMS experiment has developed a new analysis object format ("Mini-AOD") targeting approximately 10% of the size of the Run 1 AOD format. The motivation for the Mini-AOD …
GitHub - cms-ttH/MiniAOD: Tools for miniAOD exploration
Tools for miniAOD exploration. Follow These Steps: To test using the full CMSSW framework. To test using FWLite. Tools for miniAOD exploration. Contribute to cms-ttH/MiniAOD …
[PDF] Portable Acceleration of CMS Mini-AOD Production with ...
In this setup, the main CMS Mini-AOD creation workflow is executed on CPUs, while several machine learning (ML) inference tasks are offloaded onto (remote) coprocessors, such as GPUs.