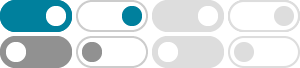
在NLP中理解CNN - 知乎 - 知乎专栏
CMU NLP公开课笔记(四)——CNN on modeling sentence. 本节课主要是讲解如何使用卷积神经网络(CNN)应用于NLP任务中,具体主要是对文本中的句子对象进行建模。虽然CNN在图像领域应用较为广泛,但是在NLP中,也不乏很多CNN的应用实例,比如文本…
NLP with CNNs | Towards Data Science
2020年10月13日 · CNNs can be used for different classification tasks in NLP. A convolution is a window that slides over a larger input data with an emphasis on a subset of the input matrix. Getting your data in the right dimensions is extremely important for any learning algorithm.
NLP经典模型系列(四):CNN - 知乎 - 知乎专栏
一、为什么可以将cnn应用于 nlp ? 作为一个非常有用的特征提取方案,CNN最初是在计算机视觉领域应用的。 个人认为,CNN在进行信息提取时,是基于一定的窗口尺寸,并且在窗口内对特征进行内积,可以提取到窗口内特征之间的融合信息,有一点类似于N-grams的 ...
【NLP】保姆级教程:手把手带你CNN文本分类(附代码)-CSDN博客
2021年9月27日 · 那么,cnn到底是怎么应用到nlp上的呢? 不同于CV输入的图像像素,NLP的输入是一个个句子或者文档。 句子或文档在输入时经过 embedding (word2vec或者Glove)会被表示成向量矩阵,其中每一行表示一个词语,行的总数是句子的长度,列的总数就是维度。
解读NLP深度学习的各类模型 - 知乎 - 知乎专栏
CNN-based Model 词向量通过低维分布式空间能够有效地表示词,使其成为NLP深度学习研究的基石。 在词向量的基础上,需要有一种有效的特征提取器从词向量序列里提取出更高层次的特征,应用到NLP任务中去,譬如机器翻译、情感分析、问答、摘要,等。
理解NLP中的卷积神经网络(CNN) - CSDN博客
2018年4月29日 · nlp课程第11讲介绍了卷积神经网络 (cnn)及模型细节,并讲解cnn和深度cnn在文本分类中的使用,最后介绍了q-rnn模型。 卷积神经网络 CNN 及其在 NLP 中应用 orangerfun的博客
2016年5月12日 · We report on a series of experiments with convolutional neural networks (CNN) trained on top of pre-trained word vec-tors for sentence-level classification tasks. We show that a simple CNN with lit-tle hyperparameter tuning and static vec-tors achieves excellent results on multi-ple benchmarks.
CNN In NLP: Top 5 Types & How To Tutorial For Text Classification
2023年1月10日 · What is a CNN in NLP? A common type of neural network used for tasks involving natural language processing is the CNN (Convolutional Neural Network). It works particularly well when dealing with data sequences, like text. CNNs can be used in NLP to perform language modelling, machine translation, and text classification.
卷积神经网络(CNN)在自然语言处理(NLP)中的应用解析
2024年3月18日 · 本文介绍了卷积神经网络(CNN)在自然语言处理(NLP)中的基本原理、应用案例及实际效果,通过生动的语言和实例,让读者轻松理解CNN在NLP中的重要作用,并提供实用的操作建议。
A Complete Guide to CNN for Sentence Classification with PyTorch
2020年2月1日 · With only a simple one-layer CNN trained on top of pretrained word vectors and little hyperparameter tuning, the model achieves excellent results on multiple sentence-level classification tasks. CNN models are now used widely in other NLP tasks such as translation and question answering as a part of a more complex architecture.