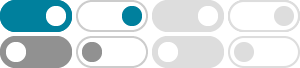
Deep separable convolutional network for remaining useful life ...
2019年12月1日 · The proposed deep separable convolutional network (DSCN) directly uses the raw multi-sensor data as inputs, getting rid of the manual feature extraction and selection. The interrelationships of different sensor data are effectively modeled in the degradation behavior learning by introducing separable convolutions.
Multitarget Robust Deep Stochastic Configuration Network …
To improve the model accuracy of deep stochastic configuration network (DSCN) in multitarget robust parameter modeling tasks, this paper presents a multitarget robust DSCN modeling method. This method expands the hidden layer output matrix by optimizing the network model structure and exploiting the correlation between multiple targets to ...
超强组合!可变形卷积+注意力机制,2024持续发力!
2024年12月5日 · 可变形条带卷积(Deformable Strip Convolution, DSCN):作为DCNv3的简化版本,通过限制变形采样内核到单轴,减少了计算负载。 可变形空间注意力(Deformable Spatial Attention, DSA):用空间注意力机制替代DCNv3中的调制掩码分支,以减少参数和内存消耗。
卷积神经网络之深度可分离卷积(Depthwise Separable …
DSC由Depthwise Convolution和Pointwise Convolution两部分构成。 Depthwise Convolution的计算非常简单,它对输入feature map的每个通道分别使用一个卷积核,然后将所有卷积核的输出再进行拼接得到它的最终输出,如图1.1中的Depthwise Convolution部分所示。 因为卷积操作的输出通道数等于卷积核的数量,而Depthwise Convolution中对每个通道只使用一个卷积核,所以单个通道在卷积操作之后的输出通道数也为1。 那么如果输入feature map的通道数为N(如图1.1所 …
Dense deep stochastic configuration network with hybrid training ...
2022年1月1日 · To solve this problem, we propose a Dense DSCN with a Hybrid Training mechanism (HT-DDSCN), which extends the network structure of the DSCN to a dense connection type and combines three typical optimisation techniques and one universal control strategy to optimise the calculation process of the output weights.
祝贺实验室博士生张成龙的论文被CCF A类中文权威期刊《计算机 …
2023年3月21日 · 深度随机配置网络 (Deep Stochastic Configuration Network,DSCN) 是一种增量式随机化学习模型,具有人为干预程度低、学习效率高和泛化能力强等优点。 但是,面向噪声数据回归与分析时,传统的 DSCN 易受到异常值影响,从而降低了模型的泛化性。
变化检测DSCN论文介绍 - CSDN博客
dscn的工作原理如下:当输入信号加在非反相端时,通过反馈电阻的作用,将一部分输出信号反馈到反相端。 对于差分输入信号, dscn 可以将其放大,并输出到负载上。
YOLOv10涨点改进:卷积魔改 | 可变形条带卷积(DSCN),魔改轻 …
2024年6月6日 · 本文介绍了yolov10的改进版,独创可变形条带卷积(dscn),作为轻量级dcnv3的替代方案,减少了计算量,提高了性能。 DSCN通过限制变形采样核在单轴上,降低了计算负荷。
基于M-estimator函数的加权深度随机配置网络-【维普期刊官网】
展开更多 深度随机配置网络 (Deep Stochastic Configuration Network,DSCN)是一种增量式随机化学习模型,具有人为干预程度低、学习效率高和泛化能力强等优点.但是,面向噪声数据回归与分析时,传统的DSCN易受到异常值影响,从而降低了模型的泛化性.因此,为提高噪声数据回归的精度和鲁棒性,提出了基于M-estimator函数的加权深度随机配置网络 (Weighted Deep Stochastic Configuration Networks,WDSCN).首先,选取Huber和Bisquare 2个常用的M-estimator函数计算样本权重,利用 …
Deep Spectral Convolution Network for HyperSpectral Unmixing
2018年6月22日 · In this paper, we propose a novel hyperspectral unmixing technique based on deep spectral convolution networks (DSCN). Particularly, three important contributions are presented throughout this paper. First, fully-connected linear operation is replaced with spectral convolutions to extract local spectral characteristics from hyperspectral ...