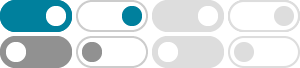
CSDN_专业开发者社区_已接入DeepSeekR1满血版
嵌入模型是将复杂数据(如文本、图像、音频等)转换为向量表示的机器学习模型,这是向量数据库能够高效处理非结构化数据的核心技术。 依此标准,万物皆可向量化,例如文字、图片、音频、视频、多模态数据,均可以有自己的标准实现向量化。 当比较标准越多,对向量的比较(相似度、区分度)也就越高,例如下图是一个高维向量。 将狗向量化,每一个比较标准即是一个向量维度,例如下图是一个二维向量。 :相似的数据在向量空间中距离相近。 Cloud_. 美团Leaf分布 …
超强组合!可变形卷积+注意力机制,2024持续发力!
2024年12月5日 · 可变形条带卷积(Deformable Strip Convolution, DSCN):作为DCNv3的简化版本,通过限制变形采样内核到单轴,减少了计算负载。 可变形空间注意力(Deformable Spatial Attention, DSA):用空间注意力机制替代DCNv3中的调制掩码分支,以减少参数和内存消耗。
Multitarget Robust Deep Stochastic Configuration Network …
To improve the model accuracy of deep stochastic configuration network (DSCN) in multitarget robust parameter modeling tasks, this paper presents a multitarget robust DSCN modeling method. This method expands the hidden layer output matrix by optimizing the network model structure and exploiting the correlation between multiple targets to ...
Welcome to the Carlson and Zelazo Lab! - University of Minnesota …
The Carlson and Zelazo Lab is located in the newly constructed Campbell Hall, home of the Institute of Child Development (ICD) at the University of Minnesota, which was founded in 1925, and continues to be one of the leading research centers in the world for inquiries into both basic and applied developmental studies.
Deep separable convolutional network for remaining useful life ...
2019年12月1日 · The proposed deep separable convolutional network (DSCN) directly uses the raw multi-sensor data as inputs, getting rid of the manual feature extraction and selection. The interrelationships of different sensor data are effectively modeled in the degradation behavior learning by introducing separable convolutions.
GitHub - hu-cv/DSCN: Online Learning Discriminative Sparse …
We propose a Discriminative Sparse Convolution Network (DSCN) for robust UAV object tracking, which introduces convolutional layers with explicit data meaning and can be learned online without using any auxiliary training data.
变化检测DSCN论文介绍 - CSDN博客
dscn是直接耦合的差分放大器,由两个输入端(非反相端和反相端)、一个输出端和一个电源端组成。 dscn 的工作原理如下:当输入信号加在非反相端时,通过反馈电阻的作用,将一部分输出信号反馈到反相端。
基于M-estimator函数的加权深度随机配置网络-【维普期刊官网】
展开更多 深度随机配置网络 (Deep Stochastic Configuration Network,DSCN)是一种增量式随机化学习模型,具有人为干预程度低、学习效率高和泛化能力强等优点.但是,面向噪声数据回归与分析时,传统的DSCN易受到异常值影响,从而降低了模型的泛化性.因此,为提高噪声数据回归的精度和鲁棒性,提出了基于M-estimator函数的加权深度随机配置网络 (Weighted Deep Stochastic Configuration Networks,WDSCN).首先,选取Huber和Bisquare 2个常用的M-estimator函数计算样本权重,利用 …
注意力机制还是太全面!结合可变形卷积直接拿下顶会!高分套路 …
2024年11月20日 · 针对轻量级卷积神经网络(cnns),提出了一种简化版的可变形卷积操作dscn,它减少了计算负载和参数数量,同时保持了dcnv3的变形采样特性。 基于DSCN,提出了一种新的注意力模块DSA,用空间注意力机制替代了DCNv3中的调制掩码分支,以减少参数和内存消 …
【论文笔记】DSCN:基于深度孪生神经网络的光学航空图像变化 …
2021年3月6日 · 本文介绍了利用深度Siamese卷积网络进行光学航空图像变化检测的方法,特别强调了孪生神经网络的结构、加权对比损失在处理样本不平衡问题上的作用,并展示了在SZTAKIAirChangeBenchmarkSet数据集上的实验结果。 本文是论文《Change Detection Based on Deep Siamese Convolutional Network for Optical Aerial Images》的阅读笔记。 根据网络的结构,现在变化检测的 模型 主要有三类,一是基于孪生 神经网络 的,二是基于伪孪生神经网络 …