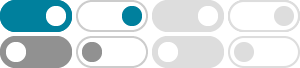
Clarify the Complexity Please! NDC Codes and Drug Classification ...
2020年4月20日 · Generic Code Number (GCN) - A GCN is a standard number assigned by a drug pricing service called First DataBank. The GCN identifies each strength, formulation, and route of administration of a drug entity.
DDI-GCN: Drug-drug interaction prediction via explainable graph ...
2023年10月1日 · DDI-GCN can help predict and understand drug-drug interactions (DDI) based on chemical structures. DDI-GCN outperformed the existing models on the prediction of both DDI outcomes and their biological consequences.
2015年1月9日 · One drug can have multiple GCNs depending on product’s available strength, forms, and route of administration. Same across all manufacturers, package size, and brand/generic products. These systems enable parties to effectively locate and identify a drug for interactions with other parties (ie. Pharmacy and their interaction with payers)
BIB | 基于注意力机制的图卷积网络预测药物-疾病关联 - 知乎
本文中作者提出了一种端到端的深度学习方法,称为LAGCN,用于预测药物与疾病的关联。 LAGCN首先将已知的药物-疾病关联、药物-药物相似性和疾病-疾病相似性整合到一个 异构网络 中,并将图卷积运算应用到网络中,学习药物和疾病的嵌入。 其次,LAGCN使用注意力机制将来自多个图卷积层的嵌入进行组合。 最后,基于整合的嵌入对未知的药物与疾病的相关性进行评分。 二、模型与方法. 2.1数据集. 作者从Comparative Toxicogenomics Database (CTD)中获取 …
Survey | 基于图卷积网络的药物发现方法 - 知乎 - 知乎专栏
GCN已被确立为与药物相关任务的最先进方法,其方式是:(1)通过考虑数据结构提取特征;(2)能够从原始输入而不是从手工制作的特征中自动提取特征这可能会导致由专家的偏见引起的重要信息。 目前正在出现的GCN遵循两个主流,可以概括为空间GCN,其通过对图中所有相邻节点的所有特征向量求和来直接在空间域中形成卷积;另一种被称为光谱GCN,根据谱图理论,它定义了图谱域中的卷积。 最近的工作还表明,谱卷积可以表征为 空间卷积 的特殊情况。 但 …
LabWeng/DDI-GCN - GitHub
2020年9月1日 · Here, we introduce DDI-GCN, which utilizes Graph Convolutional Networks (GCN) and co-attention neural networks to identify DDIs and visualize substructures that may be associated with DDIs. Besides, DDI-GCN predicts DDI types that are related to DDI pharmacology. The neural network architecture for DDI-GCN is illustrated above. DDI-GCN takes ...
A Drug-Target Interaction Prediction Based on GCN Learning
Graph Convolutional Neural Network (GCN) is one of the effective deep learning methods for complex networks. It extends the convolution operation from traditional European space to non-Euclidean space, and can simultaneously perform end-to-end node attribute information and structural information.
笔记:《Graph Convolutional Neural Networks for Predicting Drug …
2020年6月26日 · 读《Graph Convolutional Neural Networks for Predicting Drug-Target Interactions》-2019. 文章模型的目标:靶标-配体匹配,图卷积学习有意义的蛋白质口袋的框架. 提出的算法模型:用于预测蛋白质-配体相互作用的GCN。 算法性能:在常见的虚拟筛选基准数据集上获得了更好或可比的3dcnn配体评分、Autodock Vina、RF-Score和nnScore. 在GCN中,每一个 node 对应一个残基,每一个边缘表征节点之间的距离,蛋白质口袋由所有的残基空间排列而 …
Exploiting Heterogeneous Graphs with GCN for Drug Repositioning
GCN efficiently extracts node features from graphs, thereby furnishing robust data support, either directly or indirectly, for drug repositioning endeavors. Enhanced GCN algorithms have the potential to significantly improve the extraction of hidden insights pertaining to diseases and drugs within heterogeneous graphs.
DTIGCCN: Prediction of drug-target interactions based on GCN …
Drug-target interaction (DTI) prediction plays an important role in drug repositioning, drug discovery, and drug design. In recent years, some DTI prediction me.