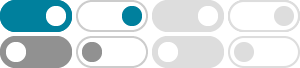
GitHub - fuxianghuang1/GH: IEEE TPAMI 2024
[IEEE TPAMI 2024] GH/GH++: Gradient Harmonization in Unsupervised Domain Adaptation The paper "Gradient Harmonization in Unsupervised Domain Adaptation" has been accepted by IEEE TPAMI 2024. Contents
Title: Gradient Harmonization in Unsupervised Domain Adaptation …
2024年8月1日 · In this paper, we delve into this issue and introduce two effective solutions known as Gradient Harmonization, including GH and GH++, to mitigate the conflict between domain alignment and classification tasks.
Gradient Harmonization in Unsupervised Domain Adaptation
2024年8月5日 · In this paper, we delve into this issue and introduce two effective solutions known as Gradient Harmonization, including GH and GH++, to mitigate the conflict between domain alignment and classification tasks.
GH/README.md at main · fuxianghuang1/GH - GitHub
[IEEE TPAMI 2024] GH/GH++: Gradient Harmonization in Unsupervised Domain Adaptation
issue and introduce two effective solutions known as Gradient Harmonization, including GH and GH++, to mitigate the conflict between domain alignment and classification tasks. GH operates by altering the gradient angle between different tasks from an obtuse angle to
Gradient Harmonization in Unsupervised Domain Adaptation
The proposed GH/GH++ is a universal plug-and-play approach for between-task balance learning and can be easily embedded in most alignment-based unsupervised domain adaptation methods. This work is a new upgrade and more general form of our previous CVPR paper from the perspective of gradient harmonization.
Domain-guided conditional diffusion model for unsupervised …
To alleviate these issues, we propose a Domain-guided Conditional Diffusion Model (DCDM), which generates high-fidelity target domain samples, making the transfer from source domain to target domain easier.
Fuxiang Huang - Papers With Code
2024年9月9日 · In this paper, we delve into this issue and introduce two effective solutions known as Gradient Harmonization, including GH and GH++, to mitigate the conflict between domain alignment and classification tasks.
Unsupervised Domain Adaptation With Hierarchical Gradient
Domain adaptation attempts to boost the performance on a target domain by borrowing knowledge from a well established source domain. To handle the distribution.
Rethinking Propagation for Unsupervised Graph Domain Adaptation
2024年3月24日 · We provide a comprehensive theoretical analysis of UGDA and derive a generalization bound for multi-layer GNNs. By formulating GNN Lipschitz for k-layer GNNs, we show that the target risk bound can be tighter by removing propagation layers in source graph and stacking multiple propagation layers in target graph.