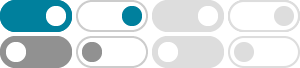
NumPy: function for simultaneous max () and min ()
2020年7月2日 · numpy.amax() will find the max value in an array, and numpy.amin() does the same for the min value. If I want to find both max and min, I have to call both functions, which requires passing over t...
python - numpy.sin function in degrees? - Stack Overflow
2015年1月22日 · I'm working on a problem that has to do with calculating angles of refraction and what not. However, it seems that I'm unable to use the numpy.sin() function in degrees. I have tried to use numpy.degrees() and numpy.rad2deg(). numpy.sin(90) numpy.degrees(numpy.sin(90)) Both return ~ 0.894 and ~ 51.2 respectively. Thanks for your …
Using Numpy Vectorize on Functions that Return Vectors
Most NumPy functions are just a bit slower than the equivalent function written in C. This is true when the NumPy function is merely a thin wrapper around a C (or Fortran) function. In contrast, a np.vectorized function still has to call a Python function once for each element in the array, so it performs more like Python code than C code ...
Are NumPy's math functions faster than Python's?
2010年9月6日 · You should use numpy function to deal with numpy's types and use regular python function to deal with regular python types. Worst performance usually occurs when mixing python builtins with numpy, because of types conversion. Those type conversion have been optimized lately, but it's still often better to not use them.
Most efficient way to map function over numpy array
2016年2月5日 · The vectorized numpy-version uses a lot of additional memory and memory-accesses. Numexp-library tries to tile the numpy-arrays and thus get a better cache utilization: # less cache misses than numpy-functionality import numexpr as ne def ne_f(x): return ne.evaluate("x+2*x*x+4*x*x*x") Leads to the following comparison:
Understanding the use of any () and all () in numpy arrays
2018年6月11日 · On 1D numpy arrays of integers like yours, any will give you True if and only if some element is non-zero, whereas all will give you True if and only if all elements are non-zero. So your first snippet of code translates into:
numpy - Integrating functions that return an array in Python
2015年12月18日 · For a vectorized but less accurate approximation, you can use numpy.trapz or scipy.integrate.simps. Your function definition (at least the one shown in the question) is implemented using numpy functions that all support broadcasting, so given a grid of x values on [0, 1], you can do this:
python - Are element-wise operations faster with NumPy …
2014年9月13日 · No, not in a significant way. The reason np.sum is faster than sum is that sum is implemented to "naively" iterate over the iterable (in this case, the numpy array), calling elements' __add__ operator (which imposes a significant overhead), while numpy's implementation of sum is optimized, e.g. taking advantage of the fact it knows the type (dtype) of the elements, and …
numpy - evaluate function on a grid of points - Stack Overflow
2014年4月1日 · What is a good way to produce a numpy array containing the values of a function evaluated on an n-dimensional grid of points? For example, suppose I want to evaluate the function defined by def f...
python - Are Numpy functions slow? - Stack Overflow
2013年8月5日 · However, when comparing Numpy ufuncs with standard Python functions I find that the latter are much faster. For example, aa = np.arange(1000000, dtype = float) %timeit np.mean(aa) # 1000 loops, best of 3: 1.15 ms per loop %timeit aa.mean # 10000000 loops, best of 3: 69.5 ns per loop I got similar results with other Numpy functions like max, power.