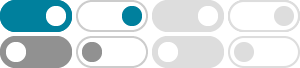
Understanding the singular value decomposition (SVD)
SVD can be applied even if the matrix is not square, unlike Eigendecomposition (another form of decomposing a matrix). SVD of any matrix A is given by: A = UDV.T (transpose of V) The matrix U and V are orthogonal matrices, D is a diagonal matrix (not necessarily square). Elements along diagonal D are known as Singular values.
To what extent is the Singular Value Decomposition unique?
2013年6月21日 · We know that the Polar Decomposition and the SVD are equivalent, but the polar decomposition is not unique unless the operator is invertible, therefore the SVD is not unique. What is the difference between these uniquenesses?
What is the intuitive relationship between SVD and PCA?
$\begingroup$ SVD and PCA and "total least-squares" (and several other names) are the same thing. It computes the orthogonal transform that decorrelates the variables and keeps the ones with the largest variance.
linear algebra - Intuitively, what is the difference between ...
2013年3月4日 · This is not much different from seeking a representative basis under which a linear transformation has its simplistic coordinate expression. Moreover, the above (incomplete) arguments showed that eigen-decomposition and SVD are closely related -- in fact, one way to derive SVD is completely from the eigen-decomposition.
Using QR algorithm to compute the SVD of a matrix
2014年3月1日 · $\begingroup$ So for finding the svd of X, we first find the Hessenberg decomposition of (XX') (let's call it H) , then using QR iteration, Q'HQ is a diagonal matrix with eigenvalues of XX' on the diagonal. Q is the matrix of eigen vectors which is equal to U of svd. then we can find V. Am I right? $\endgroup$ –
linear algebra - Full and reduced SVD of a 3x3 matrix.
2019年1月3日 · I believe that this answers both b. and c. because this is the reduced SVD and it's regarding a square matrix, so it's already a full SVD? d. and e. First I calculate the matrices and then find the determinants of the upper left principals of the matrix, if they are all non-negative numbers, they will be positive semidefinite, if the ...
Strang's proof of SVD and intuition behind matrices $U$ and $V$
2017年5月11日 · Thus the SVD produces not just the singular values and this nice factorization, but simultaneously a set of orthonormal bases for the four subspaces. Share Cite
svd - What does singular value decomposition of covariance …
Keep in mind that because all covariance matrix are symmetric and positive semi-definite, their singular values are the same as their eigenvalues. So you don't actually need to compute the SVD and can just directly compute the eigenvalues if you are interested in a rotation invariant measure of scale.
linear algebra - How to resolve the sign issue in a SVD problem ...
2017年7月16日 · The SVD transformation has an inherit ambiguity on the signs of the eigenvectors. It is not possible to solve this problem using just math. This paper , from the Sandia National Laboratores, proposes a solution to this problem by analysing the directions of the original vectors and selecting the sign of the eigenvector to be the same sign that ...
Pseudoinverse matrix and SVD - Mathematics Stack Exchange
$\begingroup$ Saying "SVD decomposition" is not quite unlike saying "enter your PIN number into the ATM machine"... $\endgroup$ – J. M. ain't a mathematician Commented Aug 3, 2011 at 8:31