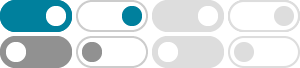
Zephyr AI
Our AI-Enabled platform leverages the combined scale and diversity of healthcare and biological data to uncover hidden insights that allow us match patients to drugs and reveal biological context that can be used to make developing new drugs faster and more efficient.
Zephyr AI
At Zephyr we're building a team of highly motivated experts who: Thrive in cross disciplinary environments Revel in the continuous cycle of learning and teaching, fostering a culture of intellectual growth Obsessively dedicate themselves to delivering transformative solutions that elevate patient outcomes
Zephyr AI
Zephyr's machine learning models seamlessly integrate clinical and molecular patient features with drug structures and targets, enabling the prediction of drug response and the identification of gene networks critical to tumor survival.
Zephyr AI
Zephyr AI Demonstrates Predictive Value of Artificial Intelligence (AI) with RWD for Label Expansion with Approved 3rd Generation EGFR Inhibitor at the 2023 ASCO Annual Meeting
Zephyr AI
Work at Zephyr AI We are a multidisciplinary team of world-class software engineers, scientists, and entrepreneurs dedicated to leveraging big data and cutting-edge technology to derive transformational insights, build enduring partnerships aimed at revolutionizing the treatment of cancer, diabetes, and other diseases.
We developed systems biology-informed AI methods trained on vast multi-omics and multi-modal datasets from DepMap, TCGA and other publicly available sources, to uncover latent vulnerabilities existing in tumors (Figure 1).
- [PDF]
2024 ASCO Poster
Zephyr has developed an AI/ML method that integrates and harnesses insights from large, diverse and disparate multi-modal datasets, to create a novel patient selection tool that uniquely advances precision medicine.
Zephyr AI Machine Learning (ML) method reconstructs transcriptomes with high accuracy across multiple tumor types Our expression reconstruction model, trained on the DepMap dataset, used 720 cell lines for training and 164 for testing.
Zephyr AI model predicts TME features associated with clinical features We next examined patterns across cancer subtypes in TCGA data and a curated real-world dataset with ICI-related patient outcomes.
SITC Poster 1295- Sunil