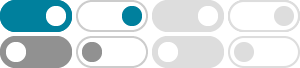
neural network - SGD versus Adam Optimization Clarification
2020年6月10日 · It states that SGD optimization updates the parameters with the same learning rate (i.e. it does not change throughout training). They state Adam is different as learning rate is variable (adaptive), and can change during training. Is this the primary difference why Adam performs (for most cases) better than SGD?
Why not always use the ADAM optimization technique?
Here’s a blog post reviewing an article claiming SGD is a better generalized adapter than ADAM. There is often a value to using more than one method (an ensemble), because every method has a weakness.
Why does Adam outperform SGD in logistic regression?
2022年11月24日 · I am training a logistic regression model. In case it matters, the features are 1376-dimensional embeddings output from a neural network. I tried both SGD and Adam with a learning rate of $10^{-3}$ for 100 epochs, and the final …
machine learning model - SGD performing better than Adam in …
2022年9月11日 · Generally the SGD optimizer uses a higher learning rate than the Adam optimizer, see for example the defaults for tensorflow (0.01 for SGD versus 0.001 for Adam). $\endgroup$ – Oxbowerce Commented Sep 11, 2022 at 17:40
Why does Faster R-CNN use SGD optimizer instead of Adam?
2020年8月27日 · In my understanding, Adam optimizer performs much better than SGD in a lot of networks. However, the paper of Faster R-CNN choose SGD optimizer instead of Adam and a lot of implementations of Faster R-CNN I found on github use SGD as optimizer as well. I guess that in case for Faster R-CNN Adam maybe doesn't have a better performance.
Policy gradient: why does this converge with Adam and not SGD?
ADAM: Adaptive Moment Estimation, this optimization algorithm take both momentum and the sum squares of the gradient is considered when calculating delta for the next iteration. SDG: Gradient Descent in TF-Keras can be implemented with momentum modification (not by default though), this is simply the previous gradient multiply by some decay rate.
machine learning - Explanations about ADAM Optimizer algorithm …
2024年8月7日 · Adam optimization is an extension of stochastic gradient descent (SGD) optimization. SGD maintains a single learning rate for all weight updates and the learning rate does not change during training. Adam optimization can have a different learning rate for each weight and change the learning rate during training.
Difference between RMSProp with momentum and Adam …
So here is another difference: The moving averages in Adam are bias-corrected, while the moving average in rmsprop with momentum is biased towards $0$. For more about the bias-correction in Adam, see section 3 in the paper and also this answer. Simulation Python Code
How similar is Adam optimization and Gradient clipping?
Adam, on the other hand, is an optimizer. It came as an improvement over RMSprop. It came as an improvement over RMSprop. The improvement was to have the goodness of both, i.e. Momentum and RMSProp ( Read this answer )
RMSProp vs Momentum in Deep Learning - Data Science Stack …
2024年8月7日 · is added to SGD() and Adam() in PyTorch. RMSProp(2012): is the optimizer which can do gradient descent by automatically adapting learning rate to parameters, considering the past and current gradients, giving much more importance to newer gradients than Momentum(1964) with EWA to accelerate convergence by mitigating fluctuation.