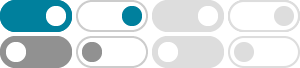
GitHub - txie-93/cgcnn: Crystal graph convolutional neural …
The package provides two major functions: Train a CGCNN model with a customized dataset. Predict material properties of new crystals with a pre-trained CGCNN model. The following paper describes the details of the CGCNN framework: Crystal Graph Convolutional Neural Networks for an Accurate and Interpretable Prediction of Material Properties
CGCNN - 知乎
在本文中,我们提出了一种广义晶体图卷积神经网络 (CGCNN)框架,用于表示周期性晶体系统,既提供了具有DFT精度的材料性质预测,又提供了原子级别的化学见解。 “深度学习”最近的进展使我们能够从非常原始的数据表示 (如图像像素)中学习,从而有可能构建优于传统专家设计的表示 [10]的通用模型。 通过研究晶体表示的最简单形式,即晶体中的原子连接,我们直接在晶体结构生成的晶体图上构建卷积神经网络。 使用Materials Project [11]的数据训练后,CGCNN对8种不 …
CGCNN 开源项目教程 - CSDN博客
2024年8月8日 · 此教程介绍了 CGCNN 开源项目的基本目录结构、启动文件及其用途。 要深入理解和使用该项目,建议直接阅读源代码并与官方文档配合理解。
[1710.10324] Crystal Graph Convolutional Neural Networks for …
2017年10月27日 · Here, we develop a crystal graph convolutional neural networks framework to directly learn material properties from the connection of atoms in the crystal, providing a universal and interpretable representation of crystalline materials.
Crystal Graph Convolutional Neural Networks for an Accurate and ...
2018年4月6日 · Here, we develop a crystal graph convolutional neural networks framework to directly learn material properties from the connection of atoms in the crystal, providing a universal and interpretable representation of crystalline materials.
CGCNN-CSDN博客
2022年8月12日 · 在本文中,我们提出了一种广义晶体 图卷积神经网络 (CGCNN)框架,用于表示周期性晶体系统,既提供了具有DFT精度的材料性质预测,又提供了原子级别的化学见解。 “ 深度学习 ”最近的进展使我们能够从非常原始的数据表示 (如图像像素)中学习,从而有可能构建优于传统专家设计的表示 [10]的通用模型。 通过研究晶体表示的最简单形式,即晶体中的原子连接,我们直接在晶体结构生成的晶体图上构建卷积神经网络。 使用Materials Project [11]的数据训练 …
GitHub - kaist-amsg/CGCNN-HD
The CGCNN-HD is a python code for dropout-based uncertainty quantification for stability prediction with CGCNN (by T. Xie et al.) developed by prof. Yousung Jung group at KAIST (contact: [email protected]).
CGCNN 项目常见问题解决方案 - CSDN博客
2024年9月13日 · CGCNN(Crystal Graph Convolutional Neural Networks)是一个开源项目,旨在通过晶体图卷积神经网络来预测材料的性质。 该项目由 Tian Xie 创建并维护,主要使用 Python 编程语言。 CGCNN 的核心功能包括训练自定义数据集的 CGCNN 模型,以及使用预训练的 CGCNN 模型来预测新晶体的材料性质。 1. 环境配置问题. 问题描述:新手在配置项目运行环境时,可能会遇到依赖库安装失败或版本不兼容的问题。 安装 Conda:首先确保你已经安装了 …
GitHub - JFLigroup/PH-CGCNN: Persistent Homology Crystal …
For training and testing, you need a dataset consisting of structure files (in CIF format) and related properties data (id_prop.csv) in the same directory (data_demo), along with a topology …
<br>用于预测高度不平衡数据的Boosting-Crystal Graph卷积神经 …
The proposed approach, termed boosting–CGCNN, combines the crystal graph convolutional neural network (CGCNN) model with a gradient-boosting algorithm. The model effectively handled extreme class imbalances in MIT material data by sequentially building a deeper neural network.