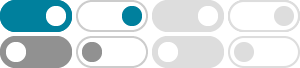
Denoising Hamiltonian Network for Physical Reasoning
2025年3月10日 · We propose the Denoising Hamiltonian Network (DHN), a novel framework that generalizes Hamiltonian mechanics operators into more flexible neural operators. DHN captures non-local temporal relationships and mitigates numerical integration errors through a …
【ECCV 2024】傅里叶卷积混合器 - CSDN博客
2024年12月25日 · FFCM:基于快速傅里叶卷积(FFC),通过空间 - 频率域的卷积操作融合多尺度空间特征,比 Transformer 中的自注意力机制更高效地提取全局信息。 PGFN:引入残差通道先验(RCP)信息,以门控方式引导前馈网络增强局部细节和结构恢复能力。
将哈密顿力学泛化到神经算子,何恺明团队又发新作,实现更高级 …
为了解决这些限制,何恺明等来自 MIT、斯坦福大学等机构的研究者提出了去噪哈密顿网络(Denoising Hamiltonian Network,DHN),这是一个将 哈密顿力学 泛化到 神经算子 的框架。
olution Mixer (FFCM) and the Prior-Gated Feed-forward Network (PGFN). In practice, FFCM, built upon Fast Fourier Convolution [6] (FFC), blends multi-scale spatial features in the frequency domain to extract global information more eficiently than the …
RT-DETR融合[ECCV2024]FADformer中的FFCM模块 - CSDN博客
2024年12月23日 · 与自注意力机制不同,FFCM 在空间域和频域中进行卷积运算,使其具有局部-全局捕获能力和效率。 同时,PGFN 以门控方式先导残基通道,以增强局部细节并保留特征结构。 此外,我们在训练过程中引入了频域对比 正则化 (FCR)。 FCR 有助于在 频域 中进行对比学习,并利用负样本中的雨纹模式来提高性能。 广泛的实验表明了我们的 FADformer 的效率和有效性。 总结:作者针对图像重建提出FADformer,其中的FFCM模块可用于增强其他模块。 ⭐⭐ …
YOLOv11融合[ECCV2024]FADformer中的FFCM模块 - CSDN博客
与自注意力机制不同,FFCM 在空间域和频域中进行卷积运算,使其具有局部-全局捕获能力和效率。 同时,PGFN 以门控方式先导残基通道,以增强局部细节并保留特征结构。 此外,我们在训练过程中引入了频域对比 正则化 (FCR)。 FCR 有助于在 频域 中进行对比学习,并利用负样本中的雨纹模式来提高性能。 广泛的实验表明了我们的 FADformer 的效率和有效性。 总结:作者针对图像重建提出FADformer,其中的FFCM模块可用于增强其他模块。 ⭐⭐ 本文二创模块仅更新 …
ECVA | European Computer Vision Association
To tackle these problems, we propose a Frequency-Aware Deraining Transformer Framework (FADformer) that fully captures frequency domain features for efficient rain removal. Specifically, we construct the FADBlock, including the Fused Fourier Convolution Mixer (FFCM) and Prior-Gated Feed-forward Network (PGFN).
Efficient Frequency-Domain Image Deraining with Contrastive ...
2025年1月12日 · 提出了一种高效的频率感知变换器架构(FADformer):该架构结合了频率域卷积混合器(FFCM)和基于先验的馈前网络(PGFN),能够在保持全局-局部特征的同时生成高质量的去雨结果。
An improved DNN with FFCM method for multimodal brain tumor ...
2023年5月1日 · In FFCM method, the number of iterations needed to converge to a solution is reduced by introducing a stopping criterion based on the sum of changes in the cluster centroids between two consecutive iterations. Further, FFCM method is chosen for segmenting tumor core and edema for its ability to handle noise and partial volume effects.
Improvement of Neural-Network Classifiers Using Fuzzy Floating ...
In this article, a fuzzy floating centroids method (FFCM) is proposed, which uses a fuzzy strategy and the concept of floating centroids to enhance the performance of the neural-network classifier. The decision boundaries in the traditional floating centroids neural-network (FCM) classifier are …
- 某些结果已被删除