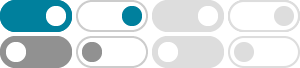
手撕 CNN 经典网络之 VGGNet(理论篇) - 知乎专栏
概括来说,VGG由5层卷积层(每个卷积层的子层数量不同)、3层全连接层、softmax输出层构成,层与层之间使用maxpooling(最大化池)分开,所有隐层的激活单元都采用ReLU函数。
GSTE: an Illustrative and comparative introduction - IEEE Xplore
GSTE (Generalized Symbolic Trajectory Evaluation) is an industrially proven high capacity model checking technology for hardware verification. It combines the efficiency and flexibility of the …
RepVGG:极简架构,SOTA性能,让VGG式模型再次伟大(CVPR …
我们最近的工作RepVGG,用 结构重参数化 (structural re-parameterization)“复兴”VGG式单路极简架构,一路3x3卷到底,在速度和性能上达到SOTA水平,在ImageNet上超过80%正确率 …
经典CNN模型(三):VGG(PyTorch详细注释版) - CSDN博客
2024年7月24日 · VGG-16 是一种深度 卷积神经网络 (CNN),由牛津大学视觉几何组(Visual Geometry Group,简称VGG)的 Karen Simonyan和Andrew Zisserman 在 2014 年提出。 …
深度学习中的经典模型:VGG详解 - CSDN博客
2025年1月19日 · VGG是一种用于图像分类的卷积神经网络(CNN)模型。 它的名字来源于提出它的研究团队——Visual Geometry Group。 VGG的核心思想是通过堆叠多个小尺寸的卷积层来 …
A Faithful Semantics for Generalised Symbolic Trajectory Evaluation
2009年1月16日 · Generalised Symbolic Trajectory Evaluation (GSTE) is a high-capacity formal verification technique for hardware. GSTE uses abstraction, meaning that details of the circuit …
GSTE through a case study | Proceedings of the 2002 IEEE/ACM ...
2002年11月10日 · We address the problem of implication of assertion graphs that occur in generalized symbolic trajectory evaluation (GSTE). GSTE has demonstrated its powerful …
[1409.1556] Very Deep Convolutional Networks for Large-Scale …
2014年9月4日 · Our main contribution is a thorough evaluation of networks of increasing depth using an architecture with very small (3x3) convolution filters, which shows that a significant …
基于GSTE模型检测的信号并串转换模块功能验证的研究-学位-万方 …
通过与基于BDD的GSTE模型检测方法的性能对比,证明了结合AIG和SAT求解器的验证方法有效地提高了验证规模,并且在验证例如SPDIF_ENCODE等规模较大电路时有更好的验证性能。
基于广义符号轨迹赋值理论的模型检测 - 百度百科
《基于广义符号轨迹赋值理论的模型检测》是依托电子科技大学,由杨国武担任醒目负责人的面上项目。 广义符号轨迹赋值(GSTE)是一种针对超大规模集成化电路设计的符号轨迹赋值的推广 …