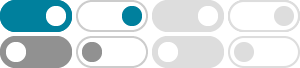
Integrated nested Laplace approximations - Wikipedia
Integrated nested Laplace approximations (INLA) is a method for approximate Bayesian inference based on Laplace's method. [1] .
in-laws 和parents-in-law有什么区别啊? - 百度知道
in-laws和parents-in-law的区别为:意思不同、用法不同、侧重点不同。 1、in-laws: (尤指)公婆。 2、parents-in-law:配偶的双亲。 1、in-laws:作名词时用于指“丈夫之父母”,即“公公婆婆”,是可数名词,用作对家人的称谓时,可视为专有名词,一般不用冠词。 也可用于比喻,一般无冠词。 2、parents-in-law:“妻子之父母”,即“岳父岳母”,用于比喻可指“根由,本源”,作此解时,其后常接of加名词或代词。 1、in-laws:姻亲 (无血缘关系的亲戚)。 2、parents-in-law:岳父岳母、 …
R-INLA Project - What is INLA?
The integrated nested Laplace approximation (INLA) is a method for approximate Bayesian inference. In the last years it has established itself as an alternative to other methods such as Markov chain Monte Carlo because of its speed and ease of use via the R-INLA package.
英文在「稱謂後方+in-law」表示「姻親關係」 - 空中美語
沒有血緣關係,而是透過婚姻結為親戚的「 姻親關係 👨👩👦」,我們可以在 稱謂的後面加上「in-law」 表示,例如:daughter-in-law 媳婦、son-in-law 女婿,此外in-law 單用時則特指「岳父/岳母」。
IN-LAW中文(简体)翻译:剑桥词典 - Cambridge Dictionary
IN-LAW翻译:姻亲。 了解更多。
Automatic MCMC sampler for hierarchical models. i. Uses Gibbs, adaptive rejection, slice sampling, or Metropolis-Hastings, depending on situation.
Introduction to INLA - Emily Wang
2020年4月14日 · In this post, I introduce the popular alternative to Bayesian MCMC - integrated nested Laplace approximations (INLA) introduced by Rue et al. (2009), with applications to generalized linear models (GLM) and specifically Bayesian negative binomial regression. © 2023 Emily Ting Wang. Powered by Jekyll & Minimal Mistakes.
IN-LAW Definition & Meaning - Merriam-Webster
2025年3月21日 · The meaning of IN-LAW is a relative by marriage.
A gentle INLA tutorial - Precision Analytics
2017年12月20日 · INLA is a fast alternative to MCMC for the general class of latent Gaussian models (LGMs). Many familiar models can be re-cast to look like LGMs, such as GLM (M)s, GAM (M)s, time series, spatial models, measurement error models, many more. To understand the gist of what INLA is doing, we need to be familiar with:
Title: Scaling Laws of Synthetic Data for Language Models - arXiv.org
6 天之前 · Large language models (LLMs) achieve strong performance across diverse tasks, largely driven by high-quality web data used in pre-training. However, recent studies indicate this data source is rapidly depleting. Synthetic data emerges as a promising alternative, but it remains unclear whether synthetic datasets exhibit predictable scalability comparable to raw pre-training data. In this work ...