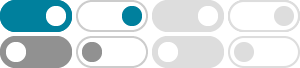
Maximum likelihood estimation - Wikipedia
In statistics, maximum likelihood estimation (MLE) is a method of estimating the parameters of an assumed probability distribution, given some observed data. This is achieved by maximizing a likelihood function so that, under the assumed statistical model, the observed data is …
最大似然估计 - 维基百科,自由的百科全书
在 统计学 中, 最大似然估计 (英語: maximum likelihood estimation,簡作 MLE),也称 极大似然估计,是用来 估計 一个 概率模型 的参数的一种方法。 下方的讨论要求读者熟悉 概率论 中的基本定义,如 概率分布 、 概率密度函数 、 随机变量 、 数学期望 等。 读者還須先熟悉 连续 实函数 的基本性质,比如使用 微分 来求一个函数的 极值 (即 极大值 或 极小值)。 同時,讀者須先擁有 似然函數 的背景知識,以了解最大似然估計的出發點及應用目的。 给定一个概率分布 , …
TMLE方法|Targeted Learning学习笔记(四) - 知乎专栏
本文将聚焦于targeted learning的核心方法, Targeted Maximum Likelihood Estimation,即 TMLE (也可以一般化称为Targeted Minimum-Loss-based Estimation,再通俗点Targeted Machine-Learning-based Estimation或许也说得通)。 从方法论上讲,TMLE是在我们的roadmap框架下前面文章中Super Learning的一个增强版;而从统计理论角度上,TMLE是 半参数方法 (semiparametric),使我们在比较弱的假定下获得相合的 (consistent)参数估计,在稍强的假定 …
Probability Density Estimation & Maximum Likelihood Estimation
2024年9月26日 · Probability Density and Maximum Likelihood Estimation (MLE) are essential tools for effectively analyzing and interpreting continuous data. The Probability Density Function (PDF) offers a clear visualization of how data points are distributed, while maximum likelihood estimation provides a robust method for estimating the parameters that best ...
Normal distribution - Maximum Likelihood Estimation - Statlect
Maximum likelihood estimation (MLE) of the parameters of the normal distribution. Derivation and properties, with detailed proofs.
Maximum Likelihood Estimation (MLE) is a widely used statistical estimation method. In this lecture, we will study its properties: efficiency, consistency and asymptotic normality. MLE is a method for estimating parameters of a statistical model.
Fitting a Model by Maximum Likelihood - R-bloggers
2013年8月18日 · Maximum-Likelihood Estimation (MLE) is a statistical technique for estimating model parameters. It basically sets out to answer the question: what model parameters are most likely to characterise a given set of data? First you need to select a model for the data. And the model must have one or more (unknown) parameters.
It is known for its signature bell curve shape, where the likelihood of a value being close to the mean is much higher than the likelihood of a value being far from the mean. The Gaussian distribution is often denoted by N(μ, σ2).
MLE of the Poisson parameter, N %&’, is the unbiased estimate of the mean, $J(sample mean)
Fitting a Model by Maximum Likelihood - datawookie.dev
2013年8月18日 · Maximum-Likelihood Estimation (MLE) is a statistical technique for estimating model parameters. It basically sets out to answer the question: what model parameters are most likely to characterise a given set of data? First you need to select a model for the data. And the model must have one or more (unknown) parameters.