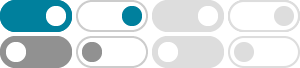
No free lunch theorem - Wikipedia
The "no free lunch" (NFL) theorem is an easily stated and easily understood consequence of theorems Wolpert and Macready actually prove. It is objectively weaker than the proven theorems, and thus does not encapsulate them.
What is No Free Lunch Theorem - GeeksforGeeks
Jun 25, 2021 · According to the “No Free Lunch” theorem, all optimization methods perform equally well when averaged over all optimization tasks without re-sampling. This fundamental theoretical notion has had the greatest impact on optimization, search, and supervised learning.
Play Online Games for Free | now.gg Mobile Cloud
Play games INSTANTLY on now.gg. Discover a world of excitement with our vast collection of free online games, conveniently accessible with no downloads required. Get ready to immerse yourself in endless fun, whether playing solo, challenging friends, or …
No Free Lunch Theorem for Machine Learning
Oct 12, 2021 · The No Free Lunch Theorem, often abbreviated as NFL or NFLT, is a theoretical finding that suggests all optimization algorithms perform equally well when their performance is averaged over all possible objective functions.
What Is the No Free Lunch Theorem? - Baeldung
Feb 28, 2025 · The No Free Lunch Theorem comes into the light to assert that we’ll fail in this approach. Instead, we should focus on finding the best possible solution for a limited scenario and input. Or maybe enhancing a previously developed model.
No free lunch theorems for optimization - IEEE Xplore
Aug 6, 2002 · A number of "no free lunch" (NFL) theorems are presented which establish that for any algorithm, any elevated performance over one class of problems is offset by performance over another class. These theorems result in a geometric interpretation of what it means for an algorithm to be well suited to an optimization problem.
Freefy - Listen to music for free
Find and listen to millions of songs, albums and artists, all completely free on .
No Free Lunch Theorems
The no free lunch theorem for search and optimization (Wolpert and Macready 1997) applies to finite spaces and algorithms that do not resample points. All algorithms that search for an extremum of a cost function perform exactly the same …
The no-free-lunch theorems of supervised learning | Synthese
The no-free-lunch theorems promote a skeptical conclusion that all possible machine learning algorithms equally lack justification. But how could this leave room for a learning theory, that shows that some algorithms are better than others?
No Free Coffee
No Free Coffee x GODZILLA VS DESTOROYAH Hoodie. $155.00 USD Quick add Quick add . No Free Coffee x GODZILLA VS MOTHRA Tee. $60.00 USD Quick add Quick add . No Free Coffee x GODZILLA VS DESTOROYAH Mug. $36.00 USD Company. About Us; Press; Support. Contact Us; Return Policy; Terms & Conditions; Join Our Community.