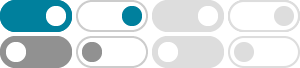
Comparing the Modeling Powers of RNN and HMM - IEEE Xplore
Recurrent Neural Networks (RNN) and Hidden Markov Models (HMM) are popular models for processing sequential data and have found many applications such as speech
Refining hidden Markov models with recurrent neural networks
Neural Computing: New Challenges and Perspectives for the New Millennium. Both hidden Markov models (HMMs) and recurrent neural networks (RNNs) have been applied to …
HMM和RNN是什么关系?功效上两者有冲突重叠? - 知乎
隐状态的表示: hmm是onehot, RNN是分布表示,RNN的表示能力强很多,或者说在面对高维度时,表示效率更高。类似nlp里,对单个token的表示,一种是onehot, 一种是word vector 。
A Hybrid RNN-HMM Approach for Weakly Supervised Temporal …
2018年12月21日 · One way to avoid frame-based human annotation is the use of action order information to learn the respective action classes. In this context, we propose a hierarchical …
(PDF) Comparing the Modeling Powers of RNN and HMM
2019年12月1日 · Recurrent Neural Networks (RNN) and Hidden Markov Models (HMM) are popular models for processing sequential data and have found many applications such as …
We finally discuss about the modeling power of the HMM and RNN submodels, via their associated observations pdf: some observations pdf can be modeled by a RNN, but not by an …
Comparing Hybrid NN-HMM and RNN for Temporal Modeling in …
2017年10月26日 · This paper provides an extended comparison of two temporal models for gesture recognition, namely Hybrid Neural Network-Hidden Markov Models (NN-HMM) and …
探索Recurrent Highway Networks:深度学习中的序列模式新星 …
2024年4月20日 · 回路高速公路网络 (RHN)的核心思想是在循环神经网络(RNN)中引入了“门控”机制,这种机制允许信息在更长时间尺度上自由流动。 与LSTM中的单元状态不同,RHN …
Modification of hybrid RNN-HMM model in asset pricing
2023年7月15日 · To further explain, this paper introduces a novel and promising hybrid RNN-HMM technique in non-categorical observations such as financial data. It seeks to combine …
Hidden Markov Model vs Recurrent Neural Network
Hidden Markov Models (HMMs) are much simpler than Recurrent Neural Networks (RNNs), and rely on strong assumptions which may not always be true. If the assumptions are true then you …