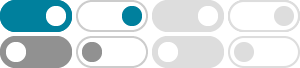
Optimal algorithm for returning top k values from an array of …
Jun 7, 2014 · At the end after you exhaust the k-2, replace the largest with -infinity and the largest of the tournament will be the kth largest. The elements you have thrown away are the top k-1 elements. This takes at most n - k + (k-1) [log (n-k+2)] comparisons to find the top k. It uses O(n) memory though.
python - Get top-k predictions from tensorflow - Stack Overflow
Jun 1, 2018 · Using tf.nn.top_k(): top_k_values, top_k_indices = tf.nn.top_k(predictions, k=k) If predictions is a vector of probabilities per class (i.e. predictions[i] = prediction probability for class i), then top_k_values will contain the k highest probabilities in predictions, and top_k_indices will contain the indices of these probabilities, i.e. the ...
python - How to calculate top-k in-class accuracies using …
Thanks, Actually I tried with following formula which gave me exact same result as Recall_k, slim.metrics.streaming_mean(tf.nn.in_top_k(predictions=logits, targets=labels, k)) I am guessing Recall_k will give me similar result as top-k accuracy.
finding top k largest keys in a dictionary python
Sep 4, 2012 · so if you want top K frequent Elements to be printed from the Dictionary; you have to use heapq.nlargest funtcion. Here is the example for the same: return heapq.nlargest(k,count.keys(), key = count.get) Here, k is the number that helps us find out elements which are repeated in a dictionary k times or more than k times.
The Most Efficient Way To Find Top K Frequent Words In A Big …
Oct 9, 2008 · Actually, we just want top K words. Other words' frequency is not concern for us. So, we can use "partial Heap sorting". For step 2) and 3), we don't just do sorting. Instead, we change it to be. 2') build a heap of (word, word-frequency) pair with "word-frequency" as key. It takes O(n) time to build a heap; 3') extract top K words from the heap.
Find the top K elements in O (N log K) time using heaps
Start with an unsorted array. Convert the first K elements to a min-heap of size K. At the top of the heap will be your smallest element. Successively replace the smallest element with each of the remaining N - K elements in the array (that do not constitute a part of the heap), in O(log K) time.
Recall, Recall rate@k and precision in top-k recommendation
Therefore, assuming user U gets a top-k recommended list of items, they would be something like: Recall= (Relevant_Items_Recommended in top-k) / (Relevant_Items) Precision= (Relevant_Items_Recommended in top-k) / (k_Items_Recommended) Until that part everything is clear but I do not understand the difference between them and Recall rate@k.
how to use tf.metrics.recall_at_k properly? - Stack Overflow
Dec 15, 2018 · If you look at open source code, you will find precision_at_k is a simple wrapper around precision_at_top_k. precision_at_k applies tf.nn.top_k first, and then calls precision_at_top_k. Documentation shows that precision_at_k expects a float tensor of logits values, but precision_at_top_k expects integer tensor the predictions to be the indices ...
How to get indices of top-K values from a numpy array
argpartition(a, k) function in numpy rearranges indices of input array a around the kth smallest element, so that all indices of smaller elements end up to the left, and all indices of bigger elements end up to the right.
python - How to find k biggest numbers from a list of n numbers ...
Jan 10, 2015 · For example the list from which I want to find k largest number be list1 > list1 = [0.5, 0.7, 0.3, 0.3, 0.3, 0.4, 0.5] Here n = 7 and if k = 3, that is if I want to find 3 largest numbers from a list of 7 numbers then output should be 0.5, 0.7, 0.5. How can this be done?